文章目录
往期文章链接目录
Sequence Data
There are many sequence data in applications. Here are some examples
- Machine translation
- from text sequence to text sequence.
- Text Summarization
- from text sequence to text sequence.
- Sentiment classification
- from text sequence to categories.
- Music Generation
- from nothing or some simple stuff (character, integer, etc) to wave sequence.
- Name entity recognition (NER)
- From text sequence to label sequence.
Why not use a standard neural network for sequence tasks
- Inputs, outputs can be different lengths in different examples. This can be solved by standard neural network by paddings with the maximum lengths but it’s not a good solution since there would be too many parameters.
- Doesn’t share features learned across different positions of text/sequence. Note that Convolutional Neural Network (CNN) is a good example of parameter sharing, so we should have a similar model for sequence data.
RNN
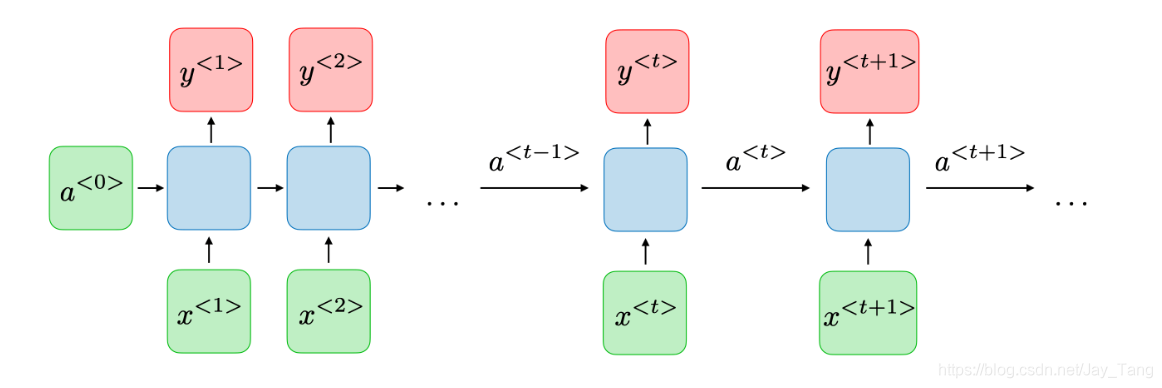
A Recurrent Neural Network (RNN) can be thought of as multiple copies of the same network, each passing a message to a successor. This chain-like nature reveals that recurrent neural networks are intimately related to sequences. Therefore, they’re the natural architecture of neural network to use for sequence data. Note that it also allows previous outputs to be used as inputs.
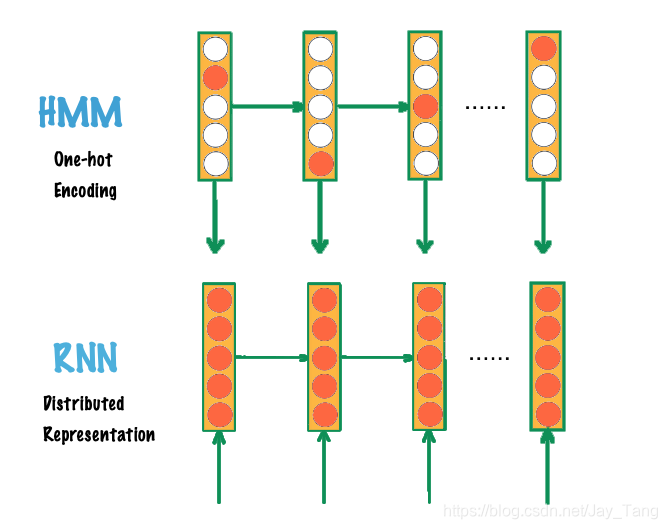
For each time step t t t, the activation a < t > a^{<t>} a<t> and the output y < t > y^{<t>} y<t> are expressed as follows:
- a < t > = g 1 ( W a a a < t − 1 > + W a x x < t > + b a ) a^{<t>}=g_{1}\left(W_{a a} a^{<t-1>}+W_{a x} x^{<t>}+b_{a}\right) a<t>=g1(Waaa<t−1>+Waxx<t>+ba)
- y < t > = g 2 ( W y a a < t > + b y ) y^{<t>}=g_{2}\left(W_{y a} a^{<t>}+b_{y}\right) y<t>=g2(Wyaa<t>+by)
These calculation could be visualized in the following figure
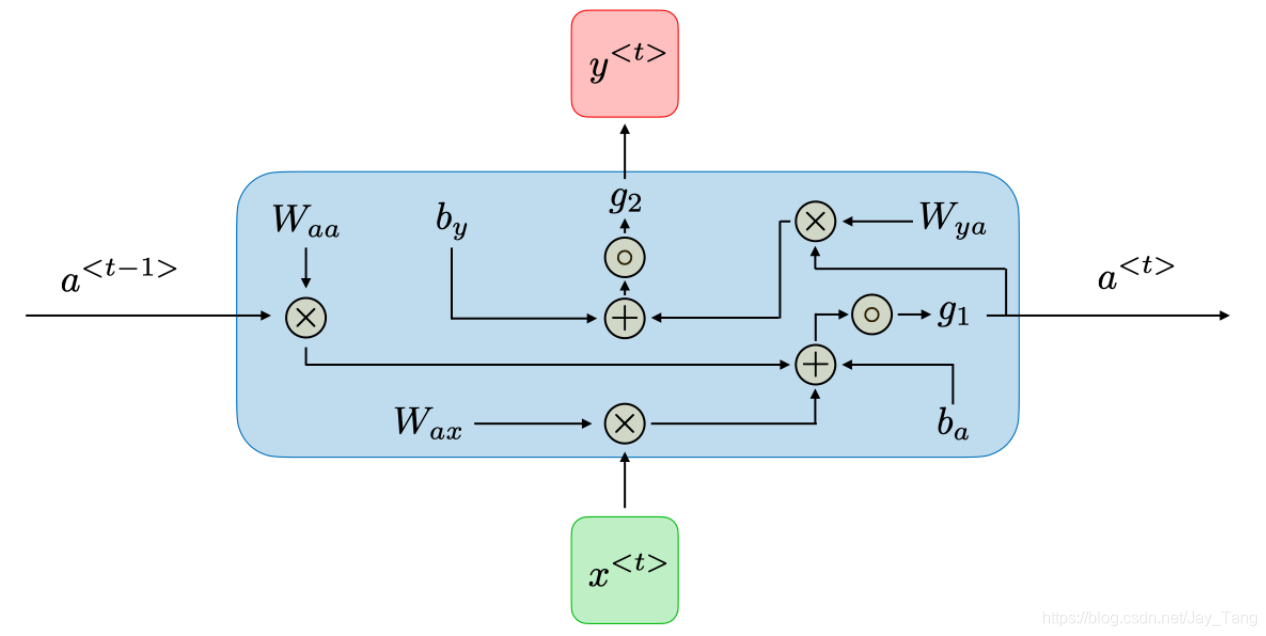
Note:
- dimension of W a a W_{a a} Waa: (number of hidden neurons, number of hidden neurons)
- dimension of W a x W_{a x} Wax: (number of hidden neurons, length of x x x)
- dimension of W y a W_{y a} Wya: (length of y y y, number of hidden neurons)
- The weight matrix W a a W_{a a} Waa is the memory the RNN is trying to maintain from the previous layers.
- dimension of b a b_a ba: (number of hidden neurons, 1)
- dimension of b y b_y by: (length of
版权声明:本文内容由互联网用户自发贡献,该文观点仅代表作者本人。本站仅提供信息存储空间服务,不拥有所有权,不承担相关法律责任。如发现本站有涉嫌侵权/违法违规的内容, 请发送邮件至 举报,一经查实,本站将立刻删除。
如需转载请保留出处:https://bianchenghao.cn/bian-cheng-ji-chu/103332.html